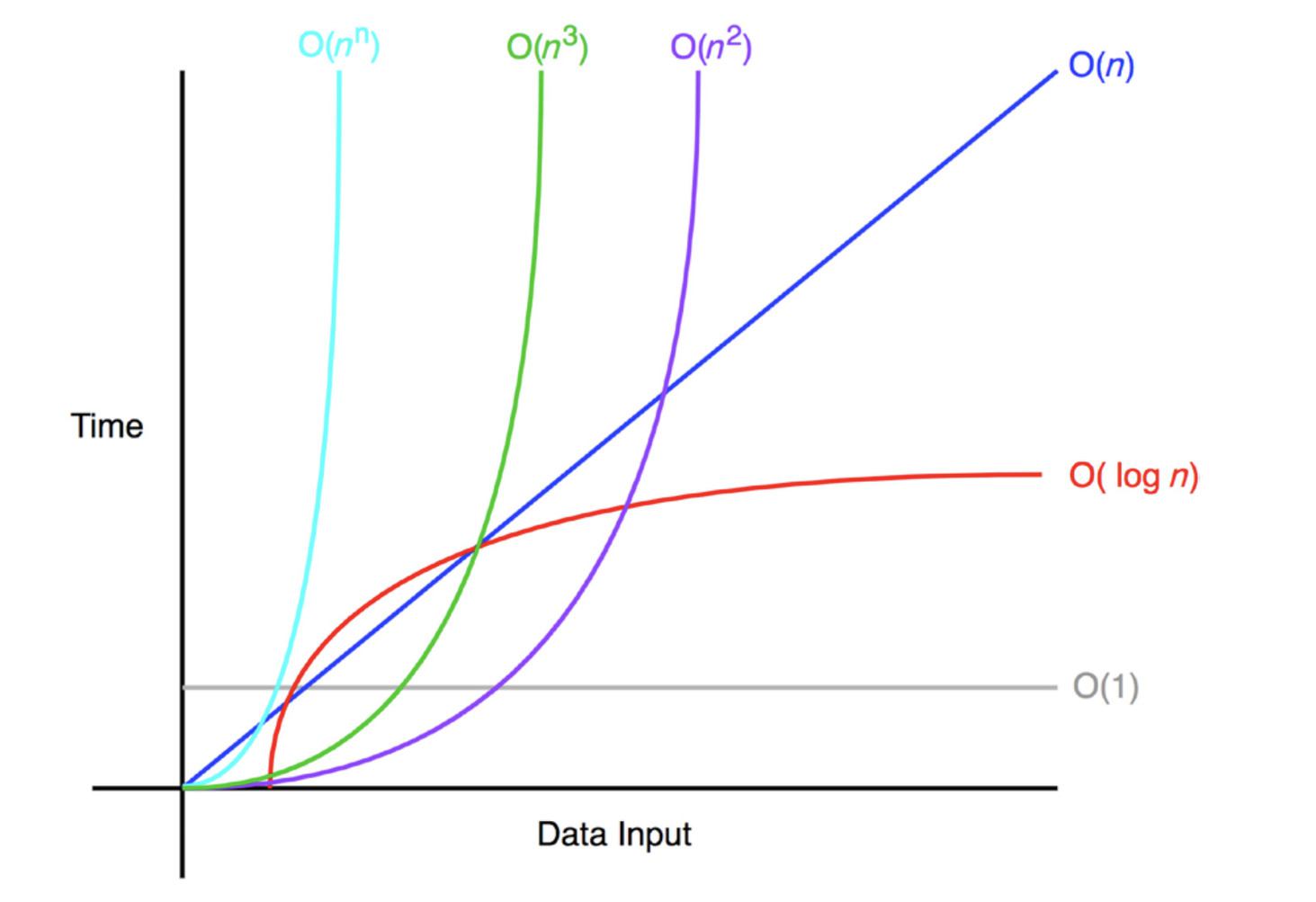
In Computer Science, Big-O is a standard mathematical notation that is used to measure the efficiency of an algorithm relative to its input size in the worst-case scenario. In other words, Big-O notation is a language that we used to measure the time or space complexity of an algorithm. To measure the efficiency of an algorithm, we need to measure the two things.
-
Time complexity:How much time is needed to run an algorithm.
-
Space Complexity:How much space is needed to run an algorithm.
Big-O notation represents the upper bound of running algorithms relative to the input size. As it represents the upper bound so it will always give you the worst-case complexity of an algorithm. It is represented as:
f(n) = O(inputSize)
How to calculate the Complexity
You need to follow below 3 steps to calculate the complexity of any program.
-
Write down each step with their execution count
-
Make an equation by summing all the step count in terms of n.
We are taking an example to understand more.
function example() { var sum = 0; for(var a=0; a<10; a++) { sum = sum + a; } console.log(sum); }
Step 1: List all the steps with their count.
Operations / Steps | Num of Executions |
---|---|
var sum = 0 |
1 |
for (int a=0;a<10;a++) |
11 |
sum = sum+a |
10 |
console.log(sum); |
1 |
Step 2: Sum all the steps
=> 1 + 11 + 10 + 1
Now generalize the above equation in terms of n (input size).
=> 1 + (n + 1) + n + 1
=> 3 + 2n
Now remove contents and ignore lower order terms to get the Big-O Notation.
=> n =>O(n)
Examples of Big-O Notation
1. Constant Time O(1): O(1) describes the complexity of the function which always executes a single time irrespective of input data set.
function constant(n) { console.log('O1'); }
2. Linear Time O(N):O(N) describes the complexity of the function which always executes n number of times where n is the number of elements in the array. If the number of elements in the array grows then the time will also grow linearly. If the value of n is 10 then the function will prints “On” 10 times.
function linear(n) { for(var a=0; a<n; a++) { console.log('On'); } }
3. Quadratic Time O(N2):O(N2) describes the complexity of the function which always executes (n*n) number of times where n is the number of elements in the array. If the number of elements in the array grows then the time will also grow quadratically.If the value of n is 10 then the function will prints “On” 100 times.
function quadratic(n) { for(var a=0; a<10; a++) { for(var a=0; a<10; a++) { console.log('On2'); } } }
Below is a rough graph of different functions to visualize their Big-o notation.
Rules of Big-O notation
We will understand the rules of Big-O notation by taking an example. Suppose we have an algorithm complexity as f(n) where n represents the number of inputs, f(n)time represents time complexing and f(n)space represents the space complexity.
Coefficient Rule: “Get Rid ofConstants”
According to this rule, we canignore any non-input-size-related constants. All the coefficients will be ignored for the large input value. So the Big-O notation of f(n) and kf(n) will be the same for any constant k > 0.
Sum Rule: “Add Big-Os Up”
The Sum rule rules stated that, If some algorithm(A) depends on two other algorithms (B+C) then the Big-O notation of algorithm (A) should be the sum of Big-O notation of Algorithm B and C.
If f(n) is O(h(n)) and g(n) is O(p(n)), then f(n)+g(n) is O(h(n)+p(n)).
Product Rule: “Multiply Big-Os”
Similar to Sum Rule, Product rule stated that Big-O notation is multiplied if the time complexity of algorithms is multiplied.
If f(n) is O(h(n)) and g(n) is O(p(n)), then f(n)g(n) is O(h(n)p(n)).
Polynomial Rule: “Big-O tothePower ofk”
The polynomial rule stated that the Big-O notation of polynomial time complexity has the same degree that of the polynomial.
If f(n) is a polynomial of degree k, then f(n) is O(nk).
Log of a power rule:
Similar to coefficients rule, constants within the log function also ignored in Big-O notation.
log(nk) is O(log(n)) for any constant k > 0.
Transitive rule:
If f(n) is O(g(n)) and g(n) is O(h(n)), then f(n) is O(h(n)).